What is Traffic Prediction? Traffic prediction is the process of forecasting traffic conditions, such as congestion and travel times, using historical traffic data.
Papers and Code
Mar 29, 2025
Abstract:Autonomous vehicle path planning has reached a stage where safety and regulatory compliance are crucial. This paper presents a new approach that integrates a motion planner with a deep reinforcement learning model to predict potential traffic rule violations. In this setup, the predictions of the critic directly affect the cost function of the motion planner, guiding the choices of the trajectory. We incorporate key interstate rules from the German Road Traffic Regulation into a rule book and use a graph-based state representation to handle complex traffic information. Our main innovation is replacing the standard actor network in an actor-critic setup with a motion planning module, which ensures both predictable trajectory generation and prevention of long-term rule violations. Experiments on an open German highway dataset show that the model can predict and prevent traffic rule violations beyond the planning horizon, significantly increasing safety in challenging traffic conditions.
* 12 pages, 7 figures. Preprint submitted to IEEE ITSC 2025
Via
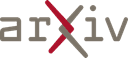
Mar 30, 2025
Abstract:With the acceleration of urbanization and the growth of transportation demands, the safety of vulnerable road users (VRUs, such as pedestrians and cyclists) in mixed traffic flows has become increasingly prominent, necessitating high-precision and diverse trajectory data to support the development and optimization of autonomous driving systems. However, existing datasets fall short in capturing the diversity and dynamics of VRU behaviors, making it difficult to meet the research demands of complex traffic environments. To address this gap, this study developed the OnSiteVRU datasets, which cover a variety of scenarios, including intersections, road segments, and urban villages. These datasets provide trajectory data for motor vehicles, electric bicycles, and human-powered bicycles, totaling approximately 17,429 trajectories with a precision of 0.04 seconds. The datasets integrate both aerial-view natural driving data and onboard real-time dynamic detection data, along with environmental information such as traffic signals, obstacles, and real-time maps, enabling a comprehensive reconstruction of interaction events. The results demonstrate that VRU\_Data outperforms traditional datasets in terms of VRU density and scene coverage, offering a more comprehensive representation of VRU behavioral characteristics. This provides critical support for traffic flow modeling, trajectory prediction, and autonomous driving virtual testing. The dataset is publicly available for download at: https://www.kaggle.com/datasets/zcyan2/mixed-traffic-trajectory-dataset-in-from-shanghai.
Via
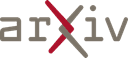
Mar 31, 2025
Abstract:Traffic engineering (TE) in large-scale computer networks has become a fundamental yet challenging problem, owing to the swift growth of global-scale cloud wide-area networks or backbone low-Earth-orbit satellite constellations. To address the scalability issue of traditional TE algorithms, learning-based approaches have been proposed, showing potential of significant efficiency improvement over state-of-the-art methods. Nevertheless, the intrinsic limitations of existing learning-based methods hinder their practical application: they are not generalizable across diverse topologies and network conditions, incur excessive training overhead, and do not respect link capacities by default. This paper proposes TELGEN, a novel TE algorithm that learns to solve TE problems efficiently in large-scale networks, while achieving superior generalizability across diverse network conditions. TELGEN is based on the novel idea of transforming the problem of "predicting the optimal TE solution" into "predicting the optimal TE algorithm", which enables TELGEN to learn and efficiently approximate the end-to-end solving process of classical optimal TE algorithms. The learned algorithm is agnostic to the exact network topology or traffic patterns, and can efficiently solve TE problems given arbitrary inputs and generalize well to unseen topologies and demands. We trained and evaluated TELGEN on random and real-world networks with up to 5000 nodes and 106 links. TELGEN achieved less than 3% optimality gap while ensuring feasibility in all cases, even when the test network had up to 20x more nodes than the largest in training. It also saved up to 84% solving time than classical optimal solver, and could reduce training time per epoch and solving time by 2-4 orders of magnitude than latest learning algorithms on the largest networks.
Via
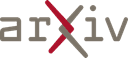
Mar 29, 2025
Abstract:Graph Neural Networks (GNNs) and differential equations (DEs) are two rapidly advancing areas of research that have shown remarkable synergy in recent years. GNNs have emerged as powerful tools for learning on graph-structured data, while differential equations provide a principled framework for modeling continuous dynamics across time and space. The intersection of these fields has led to innovative approaches that leverage the strengths of both, enabling applications in physics-informed learning, spatiotemporal modeling, and scientific computing. This survey aims to provide a comprehensive overview of the burgeoning research at the intersection of GNNs and DEs. We will categorize existing methods, discuss their underlying principles, and highlight their applications across domains such as molecular modeling, traffic prediction, and epidemic spreading. Furthermore, we identify open challenges and outline future research directions to advance this interdisciplinary field. A comprehensive paper list is provided at https://github.com/Emory-Melody/Awesome-Graph-NDEs. This survey serves as a resource for researchers and practitioners seeking to understand and contribute to the fusion of GNNs and DEs
Via
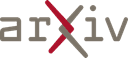
Mar 27, 2025
Abstract:Modern transportation systems face pressing challenges due to increasing demand, dynamic environments, and heterogeneous information integration. The rapid evolution of Large Language Models (LLMs) offers transformative potential to address these challenges. Extensive knowledge and high-level capabilities derived from pretraining evolve the default role of LLMs as text generators to become versatile, knowledge-driven task solvers for intelligent transportation systems. This survey first presents LLM4TR, a novel conceptual framework that systematically categorizes the roles of LLMs in transportation into four synergetic dimensions: information processors, knowledge encoders, component generators, and decision facilitators. Through a unified taxonomy, we systematically elucidate how LLMs bridge fragmented data pipelines, enhance predictive analytics, simulate human-like reasoning, and enable closed-loop interactions across sensing, learning, modeling, and managing tasks in transportation systems. For each role, our review spans diverse applications, from traffic prediction and autonomous driving to safety analytics and urban mobility optimization, highlighting how emergent capabilities of LLMs such as in-context learning and step-by-step reasoning can enhance the operation and management of transportation systems. We further curate practical guidance, including available resources and computational guidelines, to support real-world deployment. By identifying challenges in existing LLM-based solutions, this survey charts a roadmap for advancing LLM-driven transportation research, positioning LLMs as central actors in the next generation of cyber-physical-social mobility ecosystems. Online resources can be found in the project page: https://github.com/tongnie/awesome-llm4tr.
Via
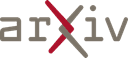
Mar 28, 2025
Abstract:The widespread adoption of Large Language Models (LLMs) has enabled diverse applications with very different latency requirements. Existing LLM serving frameworks rely on siloed infrastructure with coarse-grained workload segregation -- interactive and batch -- leading to inefficient resource utilization and limited support for fine-grained Quality-of-Service (QoS) differentiation. This results in operational inefficiencies, over-provisioning and poor load management during traffic surges. We present Niyama, a novel QoS-driven inference serving system that enables efficient co-scheduling of diverse workloads on shared infrastructure. Niyama introduces fine-grained QoS classification allowing applications to specify precise latency requirements, and dynamically adapts scheduling decisions based on real-time system state. Leveraging the predictable execution characteristics of LLM inference, Niyama implements a dynamic chunking mechanism to improve overall throughput while maintaining strict QoS guarantees. Additionally, Niyama employs a hybrid prioritization policy that balances fairness and efficiency, and employs selective request relegation that enables graceful service degradation during overload conditions. Our evaluation demonstrates that Niyama increases serving capacity by 32% compared to current siloed deployments, while maintaining QoS guarantees. Notably, under extreme load, our system reduces SLO violations by an order of magnitude compared to current strategies.
Via
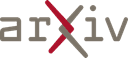
Mar 24, 2025
Abstract:The rapid increase in networked systems and data transmission requires advanced data compression solutions to optimize bandwidth utilization and enhance network performance. This study introduces a novel byte-level predictive model using Transformer architecture, capable of handling the redundancy and diversity of data types in network traffic as byte sequences. Unlike traditional methods that require separate compressors for different data types, this unified approach sets new benchmarks and simplifies predictive modeling across various data modalities such as video, audio, images, and text, by processing them at the byte level. This is achieved by predicting subsequent byte probability distributions, encoding them into a sparse rank sequence using lossless entropy coding, and significantly reducing both data size and entropy. Experimental results show that our model achieves compression ratios below 50%, while offering models of various sizes tailored for different communication devices. Additionally, we successfully deploy these models on a range of edge devices and servers, demonstrating their practical applicability and effectiveness in real-world network scenarios. This approach significantly enhances data throughput and reduces bandwidth demands, making it particularly valuable in resource-constrained environments like the Internet of Things sensor networks.
* Accepted for publication in 26th IEEE International Symposium on a
World of Wireless, Mobile and Multimedia Networks (WoWMoM)
Via
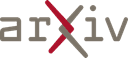
Mar 24, 2025
Abstract:The autonomous driving field has seen remarkable advancements in various topics, such as object recognition, trajectory prediction, and motion planning. However, current approaches face limitations in effectively comprehending the complex evolutions of driving scenes over time. This paper proposes FM4SU, a novel methodology for training a symbolic foundation model (FM) for scene understanding in autonomous driving. It leverages knowledge graphs (KGs) to capture sensory observation along with domain knowledge such as road topology, traffic rules, or complex interactions between traffic participants. A bird's eye view (BEV) symbolic representation is extracted from the KG for each driving scene, including the spatio-temporal information among the objects across the scenes. The BEV representation is serialized into a sequence of tokens and given to pre-trained language models (PLMs) for learning an inherent understanding of the co-occurrence among driving scene elements and generating predictions on the next scenes. We conducted a number of experiments using the nuScenes dataset and KG in various scenarios. The results demonstrate that fine-tuned models achieve significantly higher accuracy in all tasks. The fine-tuned T5 model achieved a next scene prediction accuracy of 86.7%. This paper concludes that FM4SU offers a promising foundation for developing more comprehensive models for scene understanding in autonomous driving.
Via
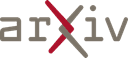
Mar 25, 2025
Abstract:Recent advancements in LLMs have revolutionized motion generation models in embodied applications. While LLM-type auto-regressive motion generation models benefit from training scalability, there remains a discrepancy between their token prediction objectives and human preferences. As a result, models pre-trained solely with token-prediction objectives often generate behaviors that deviate from what humans would prefer, making post-training preference alignment crucial for producing human-preferred motions. Unfortunately, post-training alignment requires extensive preference rankings of motions generated by the pre-trained model, which are costly to annotate, especially in multi-agent settings. Recently, there has been growing interest in leveraging pre-training demonstrations to scalably generate preference data for post-training alignment. However, these methods often adopt an adversarial assumption, treating all pre-trained model-generated samples as unpreferred examples. This adversarial approach overlooks the valuable signal provided by preference rankings among the model's own generations, ultimately reducing alignment effectiveness and potentially leading to misaligned behaviors. In this work, instead of treating all generated samples as equally bad, we leverage implicit preferences encoded in pre-training demonstrations to construct preference rankings among the pre-trained model's generations, offering more nuanced preference alignment guidance with zero human cost. We apply our approach to large-scale traffic simulation and demonstrate its effectiveness in improving the realism of pre-trained model's generated behaviors, making a lightweight 1M motion generation model comparable to SOTA large imitation-based models by relying solely on implicit feedback from pre-training demonstrations, without additional post-training human preference annotations or high computational costs.
* ICLR 2025 Spotlight
Via
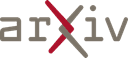
Mar 19, 2025
Abstract:The effectiveness of Spatio-temporal Graph Neural Networks (STGNNs) in time-series applications is often limited by their dependence on fixed, hand-crafted input graph structures. Motivated by insights from the Topological Data Analysis (TDA) paradigm, of which real-world data exhibits multi-scale patterns, we construct several graphs using Persistent Homology Filtration -- a mathematical framework describing the multiscale structural properties of data points. Then, we use the constructed graphs as an input to create an ensemble of Graph Neural Networks. The ensemble aggregates the signals from the individual learners via an attention-based routing mechanism, thus systematically encoding the inherent multiscale structures of data. Four different real-world experiments on seismic activity prediction and traffic forecasting (PEMS-BAY, METR-LA) demonstrate that our approach consistently outperforms single-graph baselines while providing interpretable insights.
Via
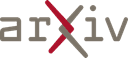